Mouse data is a subcategory of interaction data, a broad family of data about users generated as the immediate result of human interaction with computers. Its siblings from the same data family include logs of key presses or page visits. Businesses commonly rely on interaction data, including the mouse, to gather insights about their target audience. Unlike data that you could obtain more explicitly, let’s say via a survey, the advantage of interaction data is that it describes the actual behavior of actual people.
Collecting interaction data is completely unobtrusive since it can be obtained even as users go about their daily lives as usual, meaning it is a quantitative data source that scales very well. Once you start collecting it continuously as part of regular operation, you do not even need to do anything, and you’ll still have fresh, up-to-date data about users at your fingertips — potentially from your entire user base, without them even needing to know about it. Having data on specific users means that you can cater to their needs more accurately.
Of course, mouse data has its limitations. It simply cannot be obtained from people using touchscreens or those who rely on assistive tech. But if anything, that should not discourage us from using mouse data. It just illustrates that we should look for alternative methods that cater to the different ways that people interact with software. Among these, the mouse just happens to be very common.
When using the mouse, the mouse pointer is the de facto conduit for the user’s intent in a visual user interface. The mouse pointer is basically an extension of your arm that lets you interact with things in a virtual space that you cannot directly touch. Because of this, mouse interactions tend to be data-intensive. Even the simple mouse action of moving the pointer to an area and clicking it can yield a significant amount of data.
Mouse data is granular, even when compared with other sources of interaction data, such as the history of visited pages. However, with machine learning, it is possible to investigate jumbles of complicated data and uncover a variety of complex behavioral patterns. It can reveal more about the user holding the mouse without needing to provide any more information explicitly than normal.
For starters, let us venture into what kind of information can be obtained by processing mouse interaction data.
What Are Mouse Dynamics?
Mouse dynamics refer to the features that can be extracted from raw mouse data to describe the user’s operation of a mouse. Mouse data by itself corresponds with the simple mechanics of mouse controls. It consists of mouse events: the X and Y coordinates of the cursor on the screen, mouse button presses, and scrolling, each dated with a timestamp. Despite the innate simplicity of the mouse events themselves, the mouse dynamics using them as building blocks can capture user’s behavior from a diverse and emergently complex variety of perspectives.
If you are concerned about user privacy, as well you should be, mouse dynamics are also your friend. For the calculation of mouse dynamics to work, raw mouse data does not need to inherently contain any details about the actual meaning of the interaction. Without the context of what the user saw as they moved their pointer around and clicked, the data is quite safe and harmless.
Some examples of mouse dynamics include measuring the velocity and the acceleration at which the mouse cursor is moving or describing how direct or jittery the mouse trajectories are. Another example is whether the user presses and lets go of the primary mouse button quickly or whether there is a longer pause before they release their press. Four categories of over twenty base measures can be identified: temporal, spatial, spatial-temporal, and performance. Features do not need to be just metrics either, with other approaches using a time series of mouse events.
Temporal mouse dynamics:
-
Movement duration: The time between two clicks;
-
Response time: The time it takes to click something in response to a stimulus (e.g., from the moment when a page is displayed);
-
Initiation time: The time it takes from an initial stimulus for the cursor to start moving;
-
Pause time: The time measuring the cursor’s period of idleness.
Spatial mouse dynamics:
-
Distance: Length of the path traversed on the screen;
-
Straightness: The ratio between the traversed path and the optimal direct path;
-
Path deviation: Perpendicular distance of the traversed path from the optimal path;
-
Path crossing: Counted instances of the traversed and optimal path intersecting;
-
Jitter: The ratio of the traversed path length to its smoothed version;
-
Angle: The direction of movement;
-
Flips: Counted instances of change in direction;
-
Curvature: Change in angle over distance;
-
Inflection points: Counted instances of change in curvature.
Spatial-temporal mouse dynamics:
-
Velocity: Change of distance over time;
-
Acceleration: Change of velocity over time;
-
Jerk: Change of acceleration over time;
-
Snap: Change in jerk over time;
-
Angular velocity: Change in angle over time.
Performance mouse dynamics:
-
Clicks: The number of mouse button events pressing down or up;
-
Hold time: Time between mouse down and up events;
-
Click error: Length of the distance between the clicked point and the correct user task solution;
-
Time to click: Time between the hover event on the clicked point and the click event;
-
Scroll: Distance scrolled on the screen.
Note: For detailed coverage of varied mouse dynamics and their extraction, see the paper “Is mouse dynamics information credible for user behavior research? An empirical investigation.”
The spatial angular measures cited above are a good example of how the calculation of specific mouse dynamics can work. The direction angle of the movements between points A and B is the angle between the vector AB and the horizontal X axis. Then, the curvature angle in a sequence of points ABC is the angle between vectors AB and BC. Curvature distance can be defined as the ratio of the distance between points A and C and the perpendicular distance between point B and line AC. (Definitions sourced from the paper “An efficient user verification system via mouse movements.”)
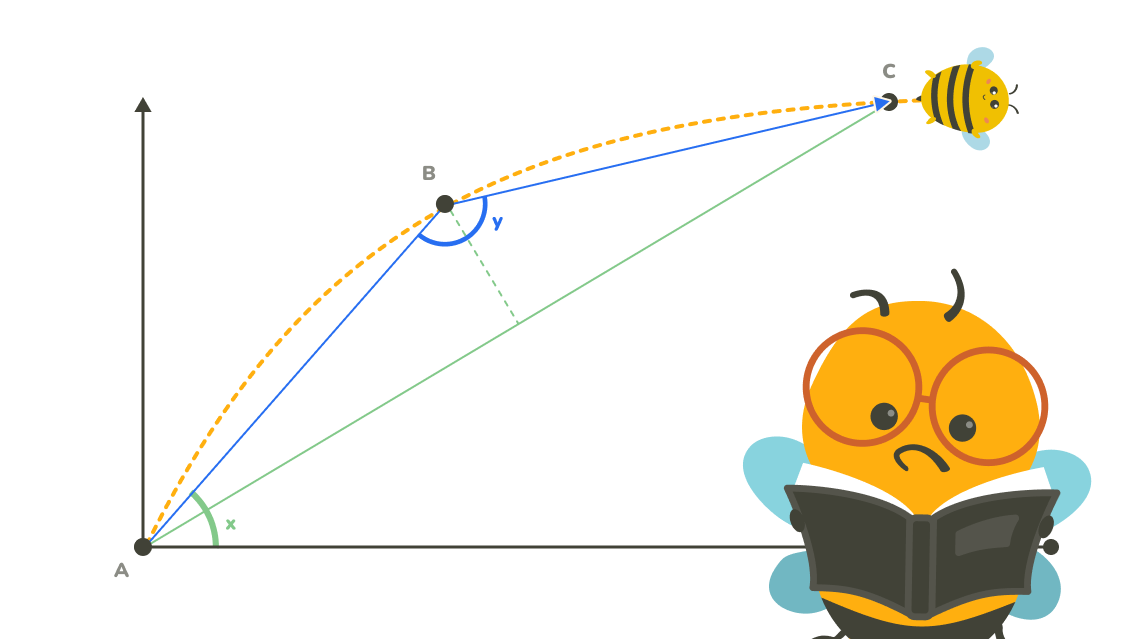
Even individual features (e.g., mouse velocity by itself) can be delved into deeper. For example, on pages with a lot of scrolling, horizontal mouse velocity along the X-axis may be more indicative of something capturing the user’s attention than velocity calculated from direct point-to-point (Euclidean) distance in the screen’s 2D space. The maximum velocity may be a good indicator of anomalies, such as user frustration, while the mean or median may tell you more about the user as a person.
From Data To Tangible Value
The introduction of mouse dynamics above, of course, is an oversimplification for illustrative purposes. Just by looking at the physical and geometrical measurements of users’ mouse trajectories, you cannot yet tell much about the user. That is the job of the machine learning algorithm. Even features that may seem intuitively useful to you as a human (see examples cited at the end of the previous section) can prove to be of low or zero value for a machine-learning algorithm.
Meanwhile, a deceptively generic or simplistic feature may turn out unexpectedly quite useful. This is why it is important to couple broad feature generation with a good feature selection method, narrowing the dimensionality of the model down to the mouse dynamics that help you achieve good accuracy without overfitting. Some feature selection techniques are embedded directly into machine learning methods (e.g., LASSO, decision trees) while others can be used as a preliminary filter (e.g., ranking features by significance assessed via a statistical test).
As we can see, there is a sequential process to transforming mouse data into mouse dynamics, into a well-tuned machine learning model to field its predictions, and into an applicable solution that generates value for you and your organization. This can be visualized as the pipeline below.
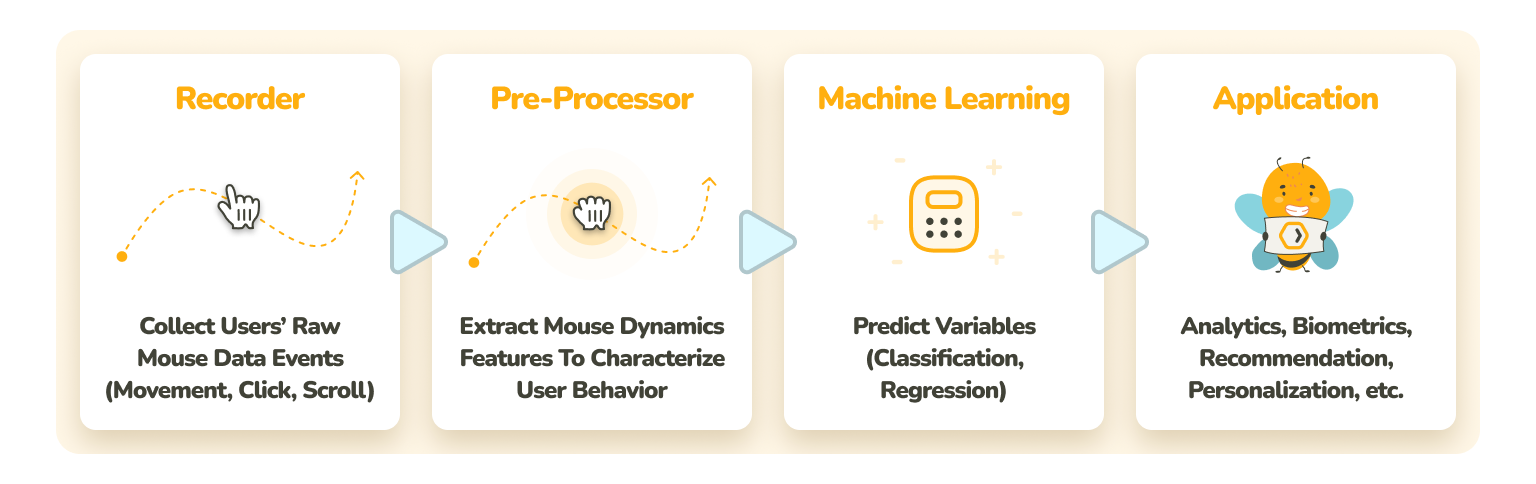
Machine Learning Applications Of Mouse Dynamics
To set the stage, we must realize that companies aren’t really known for letting go of their competitive advantage by divulging the ins and outs of what they do with the data available to them. This is especially true when it comes to tech giants with access to potentially some of the most interesting datasets on the planet (including mouse interaction data), such as Google, Amazon, Apple, Meta, or Microsoft. Still, recording mouse data is known to be a common practice.
With a bit of grit, you can find some striking examples of the use of mouse dynamics, not to mention a surprising versatility in techniques. For instance, have you ever visited an e-commerce site just to see it recommend something specific to you, such as a gendered line of cosmetics — all the while, you never submitted any information about your sex or gender anywhere explicitly?
Mouse data transcends its obvious applications, as is replaying the user’s session and highlighting which visual elements people interact with. A surprising amount of internal and external factors that shape our behavior are reflected in data as subtle indicators and can thus be predicted.
Let’s take a look at some further applications. Starting some simple categorization of users.
Example 1: Biological Sex Prediction
For businesses, knowing users well allows them to provide accurate recommendations and personalization in all sorts of ways, opening the gates for higher customer satisfaction, retention, and average order value. By itself, the prediction of user characteristics, such as gender, isn’t anything new. The reason for basing it on mouse dynamics, however, is that mouse data is generated virtually by the truckload. With that, you will have enough data to start making accurate predictions very early.
If you waited for higher-level interactions, such as which products the user visited or what they typed into the search bar, by the time you’d have enough data, the user may have already placed an order or, even worse, left unsatisfied.
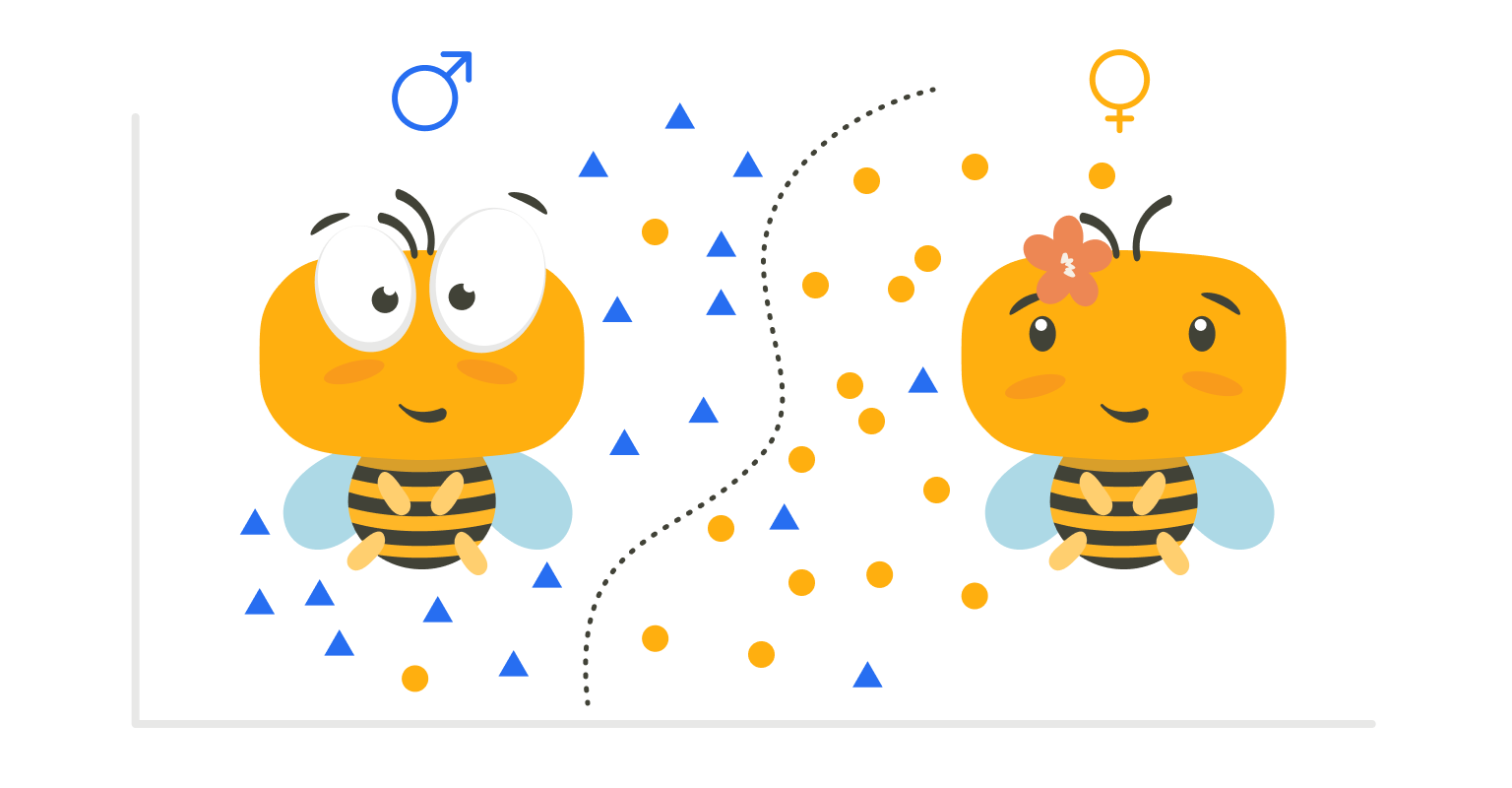
The selection of the machine learning algorithm matters for a problem. In one published scientific paper, six various models have been compared for the prediction of biological gender using mouse dynamics. The dataset for the development and evaluation of the models provides mouse dynamics from participants moving the cursor in a broad range of trajectory lengths and directions. Among the evaluated models — Logistic regression, Support vector machine, Random forest, XGBoost, CatBoost, and LightGBM — CatBoost achieved the best F1 score.
Putting people into boxes is far from everything that can be done with mouse dynamics, though. Let’s take a look at a potentially more exciting use case — trying to predict the future.
Example 2: Purchase Prediction
Another e-commerce application predicts whether the user has the intent to make a purchase or even whether they are likely to become a repeat customer. Utilizing such predictions, businesses can adapt personalized sales and marketing tactics to be more effective and efficient, for example, by catering more to likely purchasers to increase their value — or the opposite, which is investigating unlikely purchasers to find ways to turn them into likely ones.
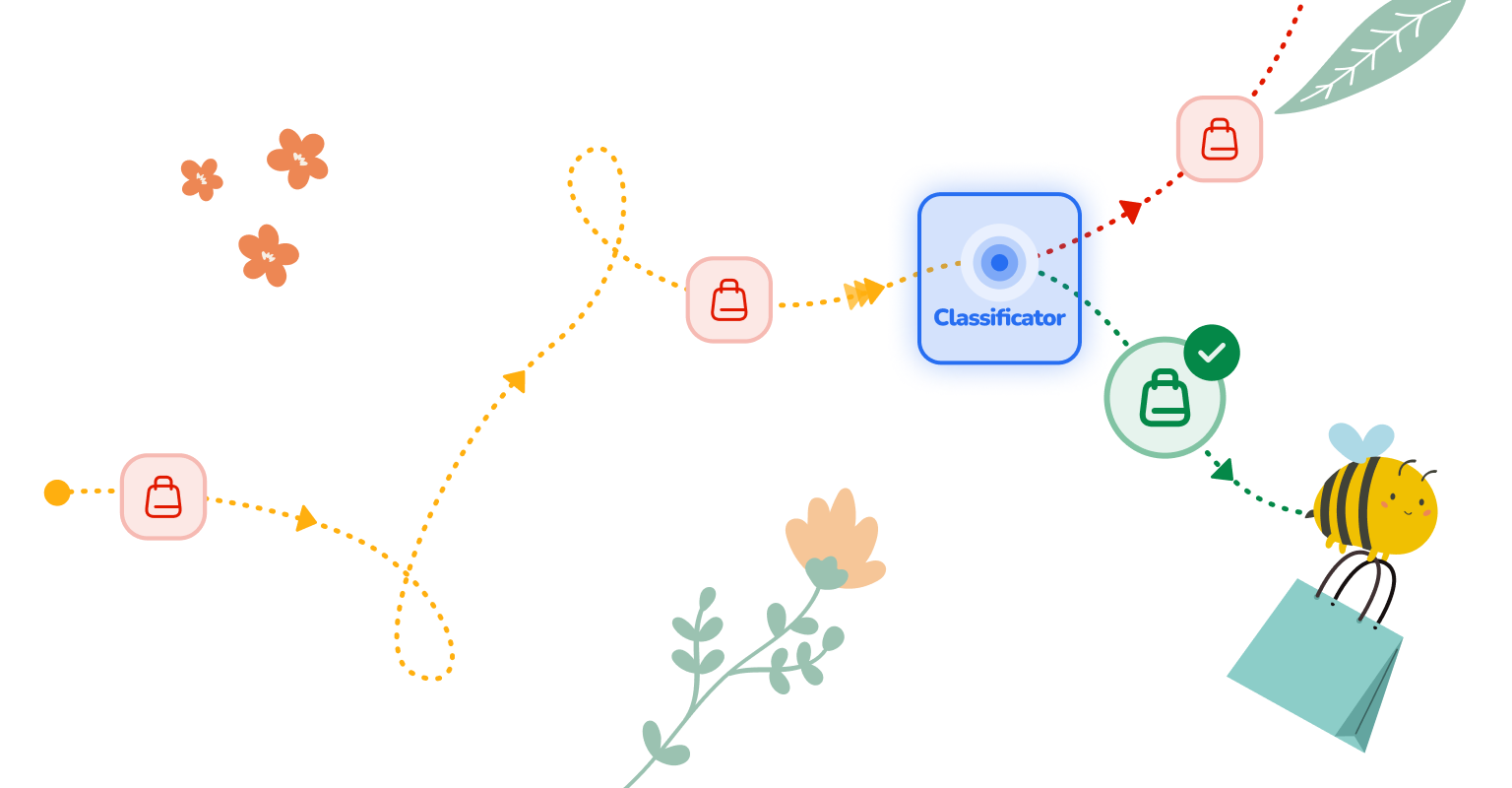
Interestingly, a paper dedicated to the prediction of repeat customership reports that when a gradient boosting model is validated on data obtained from a completely different online store than where it was trained and tuned, it still achieves respectable performance in the prediction of repeat purchases with a combination of mouse dynamics and other interaction and non-interaction features.
It is plausible that though machine-learning applications tend to be highly domain-specific, some models could be used as a starting seed, carried over between domains, especially while still waiting for user data to materialize.
Additional Examples
Applications of mouse dynamics are a lot more far-reaching than just the domain of e-commerce. To give you some ideas, here are a couple of other variables that have been predicted with mouse dynamics:
The Mouse-Shaped Caveat
When you think about mouse dynamics in-depth, some questions will invariably start to emerge. The user isn’t the only variable that could determine what mouse data looks like. What about the mouse itself?
Many brands and models are available for purchase to people worldwide. Their technical specifications deviate in attributes such as resolution (measured in DPI or, more accurately, CPI), weight, polling rate, and tracking speed. Some mouse devices have multiple profile settings that can be swapped between at will. For instance, the common CPI of an office mouse is around 800-1,600, while a gaming mouse can go to extremes, from 100 to 42,000. To complicate things further, the operating system has its own mouse settings, such as sensitivity and acceleration. Even the surface beneath the mouse can differ in its friction and optical properties.
Can we be sure that mouse data is reliable, given that basically everyone potentially works under different mouse conditions?
For the sake of argument, let’s say that as a part of a web app you’re developing, you implement biometric authentication with mouse dynamics as a security feature. You sell it by telling customers that this form of auth is capable of catching attackers who try to meddle in a tab that somebody in the customer’s organization left open on an unlocked computer. Recognizing the intruder, the app can sign the user out of the account and trigger a warning sent to the company. Kicking out the real authorized user and sounding the alarm just because somebody bought a new mouse would not be a good look. Recalibration to the new mouse would also produce friction. Some people like to change their mouse sensitivity or use different computers quite often, so frequent calibration could potentially present a critical flaw.
We found that up until now, there was barely anything written about whether or how mouse configuration affects mouse dynamics. By mouse configuration, we refer to all properties of the environment that could impact mouse behavior, including both hardware and software.
From the authors of papers and articles about mouse dynamics, there is barely a mention of mouse devices and settings involved in development and testing. This could be seen as concerning. Though hypothetically, there might not be an actual reason for concern, that is exactly the problem. There was just not even enough information to make a judgment on whether mouse configuration matters or not. This question is what drove the study conducted by UXtweak Research (as covered in the peer-reviewed paper in Computer Standards & Interfaces).
The quick answer? Mouse configuration does detrimentally affect mouse dynamics. How?
- It may cause the majority of mouse dynamics values to change in a statistically significant way between different mouse configurations.
- It may lower the prediction performance of a machine learning model if it was trained on a different set of mouse configurations than it was tested on.
It is not automatically guaranteed that prediction based on mouse dynamics will work equally well for people on different devices. Even the same person making the exact same mouse movements does not necessarily produce the same mouse dynamics if you give them a different mouse or change their settings.
We cannot say for certain how big an impact mouse configuration can have in a specific instance. For the problem that you are trying to solve (specific domain, machine learning model, audience), the impact could be big, or it could be negligible. But to be sure, it should definitely receive attention. After all, even a deceptively small percentage of improvement in prediction performance can translate to thousands of satisfied users.
Tackling Mouse Device Variability
Knowledge is half the battle, and so it is also with the realization that mouse configuration is not something that can be just ignored when working with mouse dynamics. You can perform tests to evaluate the size of the effect that mouse configuration has on your model’s performance. If, in some configurations, the number of false positives and false negatives rises above levels that you are willing to tolerate, you can start looking for potential solutions by tweaking your prediction model.
Because of the potential variability in real-world conditions, differences between mouse configurations can be seen as a concern. Of course, if you can rely on controlled conditions (such as in apps only accessible via standardized kiosks or company-issued computers and mouse devices where all system mouse settings are locked), you can avoid the concern altogether. Given that the training dataset uses the same mouse configuration as the configuration used in production, that is. Otherwise, that may be something new for you to optimize.
Some predicted variables can be observed repeatedly from the same user (e.g., emotional state or intent to make a purchase). In the case of these variables, to mitigate the problem of different users utilizing different mouse configurations, it would be possible to build personalized models trained and tuned on the data from the individual user and the mouse configurations they normally use. You also could try to normalize mouse dynamics by adjusting them to the specific user’s “normal” mouse behavior. The challenge is how to accurately establish normality. Note that this still doesn’t address situations when the user changes their mouse or settings.
Where To Take It From Here
So, we arrive at the point where we discuss the next steps for anyone who can’t wait to apply mouse dynamics to machine learning purposes of their own. For web-based solutions, you can start by looking at MouseEvents in JavaScript, which is how you’ll obtain the elementary mouse data necessary.
Mouse events will serve as the base for calculating mouse dynamics and the features in your model. Pick any that you think could be relevant to the problem you are trying to solve (see our list above, but don’t be afraid to design your own features). Don’t forget that you can also combine mouse dynamics with domain and application-specific features.
Problem awareness is key to designing the right solutions. Is your prediction problem within-subject or between-subject? A classification or a regression? Should you use the same model for your whole audience, or could it be more effective to tailor separate models to the specifics of different user segments?
For example, the mouse behavior of freshly registered users may differ from that of regular users, so you may want to divide them up. From there, you can consider the suitable machine/deep learning algorithm. For binary classification, a Support vector machine, Logistic regression, or a Random Forest could do the job. To delve into more complex patterns, you may wish to reach for a Neural network.
Of course, the best way to uncover which machine/deep learning algorithm works best for your problem is to experiment. Most importantly, don’t give up if you don’t succeed at first. You may need to go back to the drawing board a few times to reconsider your feature engineering, expand your dataset, validate your data, or tune the hyperparameters.
Conclusion
With the ongoing trend of more and more online traffic coming from mobile devices, some futurist voices in tech might have you believe that “the computer mouse is dead”. Nevertheless, those voices have been greatly exaggerated. One look at statistics reveals that while mobile devices are excessively popular, the desktop computer and the computer mouse are not going anywhere anytime soon.
Classifying users as either mobile or desktop is a false dichotomy. Some people prefer the desktop computer for tasks that call for exact controls while interacting with complex information. Working, trading, shopping, or managing finances — all, coincidentally, are tasks with a good amount of importance in people’s lives.
To wrap things up, mouse data can be a powerful information source for improving digital products and services and getting yourself a headway against the competition. Advantageously, data for mouse dynamics does not need to involve anything sensitive or in breach of the user’s privacy. Even without identifying the person, machine learning with mouse dynamics can shine a light on the user, letting you serve them more proper personalization and recommendations, even when other data is sparse. Other uses include biometrics and analytics.
Do not underestimate the impact of differences in mouse devices and settings, and you may arrive at useful and innovative mouse-dynamics-driven solutions to help you stand out.